Table of Contents
Guesswork has no place in supply chain management. A business that fails to predict demand risks stockouts, overstocking, and lost revenue.
Demand forecasting solves this by analyzing historical data, market trends, and external factors. Using it, businesses can better plan their inventory and meet customer demands.
This blog will explore why demand forecasting matters, the techniques used in supply chain management, and why AI-powered demand forecasting is the future.
What is demand forecasting in supply chain management?
Running a business without knowing how much of your product customers will buy next month is a recipe for disaster. You might overstock, wasting money on storage. Or you might understock, frustrating customers and losing sales. That’s why demand forecasting is critical.
At its core, demand forecasting is the science and art of predicting future demand for a product or service. It blends historical data, market trends, statistical models, and external factors like economic conditions or seasonality to estimate how much of a product will be needed in a given timeframe.
In supply chains, accuracy is everything. The better the forecast, the smoother the supply chain.
Why is demand forecasting important for supply chains?
Customers walking into a store, searching for their favorite product, only to find the shelf empty is bad business for the store owner. A warehouse overflowing with goods that no one wants to buy is even worse.
Demand forecasting helps companies avoid both problems. It ensures the right products are available at the right time, in the right quantity. No more guessing. No more wasted money.
Here’s a closer look at how demand forecasting benefits supply chains:
- Optimized inventory management: Helps avoid overstocking (which ties up capital) and stockouts (which lead to lost sales). Using demand forecasting ensures the right products are available at the right time, minimizing waste and maximizing profitability
- Cost reduction: With precise demand predictions, companies can optimize production schedules, reduce storage costs, and minimize wastage. This leads to lower operational expenses, saving a company sizable money in the long-term
- Better supplier and production planning: Forecasting enables businesses to align raw material procurement and manufacturing processes with expected demand, preventing bottlenecks and inefficiencies
- Enhanced customer satisfaction: Meeting customer expectations through timely order fulfillment leads to improved customer retention and brand loyalty
- Efficient resource allocation Businesses can allocate labor, transportation, and financial resources more effectively when they have a clear idea of future demand
- Improved financial planning: Accurate demand forecasts help companies make informed decisions about budgeting, pricing strategies, and revenue projections
- Risk mitigation: By analyzing past trends and market conditions, businesses can reduce uncertainty and disruptions. This happens because they can better anticipate demand fluctuations, economic downturns, or seasonal changes
Common methods for creating demand forecasts:
Businesses rely on demand forecasting to optimize inventory levels, reduce costs, and improve operational efficiency. But no single method guarantees perfect predictions.
That’s why supply chain managers use multiple forecasting techniques such as qualitative insights from industry experts and quantitative models like time series analysis to refine their estimates.
Here’s a closer look at both types of forecasting methods:
1. Quantitative forecasting
Quantitative forecasting relies on historical data. This includes customer demand patterns, supply chain performance metrics, seasonal fluctuations, and other measurable indicators.
Common methods include:
- Barometric forecasting: Utilizes current data to predict future demand through statistical analysis.
- Trend projection: Analyzes historical growth patterns to forecast sales, suitable for short-term predictions but potentially risky for long-term planning without considering additional factors.
- Exponential smoothing: Incorporates historical data and accounts for seasonal variations, beneficial for startups due to its applicability with limited datasets.
- Regression analysis: Ranges from simple to complex models, integrating internal and external data for comprehensive analysis.
- Econometric forecasting: Examines the relationship between demand data and external factors, requiring advanced statistical techniques but potentially offering more accurate forecasts.
2. Qualitative forecasting
Qualitative forecasting depends less on numerical data and more on human insights. It leverages internal expertise and external opinions. Common methods include:
- Sales force composite: Gathers insights from sales team members who interact closely with customers and can detect emerging trends early.
- Market research: Employs data on market trends and opportunities, particularly useful for startups lacking historical data.
- Delphi method: Assembles a panel of experts to iteratively answer questions, refining forecasts through consensus.
Each method has its strengths and limitations. Use both quantitative and qualitative approaches to achieve the most accurate forecasts.
Traditional vs AI demand forecasting: What’s the difference?
Traditional demand forecasting methods often rely heavily on historical data. It assumes that past patterns will continue unchanged. This approach can overlook dynamic market factors such as evolving customer behavior and economic shifts, leading to less accurate predictions.
Traditional methods work, until they don’t. Consumer behavior shifts. Markets fluctuate. Old models struggle to keep up. That’s where AI steps in.
AI-driven demand forecasting employs advanced ML algorithms capable of processing vast and diverse datasets. It analyzes historical sales alongside seasonal variations, economic indicators, competitor activities, and real-time input. This results in precise and responsive forecasts.
Here’s how traditional forecasting and AI demand forecasting are different:
Considered in the context of the supply chain industry, traditional models have proven inadequate in the face of rapidly changing consumer behaviors and market volatility. Using AI, businesses can enhance supply chain efficiency, reduce costs, minimize errors, and improve customer satisfaction.
Use cases of AI demand forecasting
Artificial Intelligence (AI) is revolutionizing demand forecasting across various industries, enhancing accuracy and operational efficiency.
Here's an in-depth look at how AI-driven demand forecasting is applied in different sectors:
1. Retail
In the retail sector, AI-driven demand forecasting is transforming operations by enhancing inventory management, personalizing customer experiences, and optimizing pricing strategies.
- Personalized recommendations: By examining customer behavior and preferences, AI tailors product suggestions, boosting sales and customer satisfaction
- Dynamic pricing: AI adjusts prices in real-time based on demand fluctuations and market trends, maximizing revenue and competitiveness
- Inventory optimization: AI analyzes historical sales data and external factors to predict demand accurately, reducing instances of stockouts and overstocking
A real-world example is Zara’s demand forecasting model. The company uses real-time sales data and AI-driven analytics to predict which clothing styles will sell best.
Instead of producing massive stock in advance, it manufactures in smaller batches and adjusts production based on demand signals from stores. This strategy reduces unsold inventory, minimizes waste and ensures that popular products are always available.
2. Supply chain management
AI enhances supply chain efficiency by providing precise demand forecasts, which inform production planning, logistics, and resource allocation.
- Logistics optimization: AI-driven forecasts assist in route planning and capacity utilization, reducing transportation costs and improving delivery times
- Supplier management: By anticipating demand, companies can negotiate better terms with suppliers and maintain optimal inventory levels
- Production planning: Accurate demand predictions enable manufacturers to align production schedules with market needs, minimizing waste and optimizing resource use
For example, Amazon uses AI-driven demand forecasting to predict product demand across its vast global network. By analyzing purchase history, seasonal trends, and real-time shopping behavior, Amazon ensures that its fulfillment centers are stocked with the right products.
This reduces delivery times, minimizes overstocking, and optimizes storage costs, enabling faster and more efficient supply chain operations.
3. Manufacturing
In manufacturing, AI-driven demand forecasting streamlines operations and reduces costs.
- Resource allocation: AI forecasts help in allocating materials and labor efficiently, ensuring production meets demand without overextending resources
- Quality control: AI identifies patterns that may lead to defects, enabling proactive measures to maintain product standards
- Maintenance scheduling: Predictive analytics anticipate equipment needs, reducing downtime and maintaining consistent production flow
Tesla leverages demand forecasting to align its electric vehicle production with market demand. By analyzing reservation data, supply chain constraints, and global EV adoption trends, Tesla adjusts manufacturing output, ensuring efficient resource utilization.
4. Finance
Financial institutions leverage AI for market analysis and risk management.
- Risk assessment: By analyzing borrower data, AI evaluates credit risk, informing lending practices and reducing default rates
- Fraud detection: AI monitors transactions in real-time, identifying anomalies that may indicate fraudulent activity
- Market trend analysis: AI examines economic indicators and historical data to forecast market movements, aiding in investment decisions
Hedge funds use demand forecasting to anticipate stock price movements. They employ machine learning algorithms to analyze historical stock trends, economic indicators, and investor sentiment. These forecasts inform trading strategies and maximize returns.
5. Healthcare
AI-driven demand forecasting in healthcare improves patient care and operational efficiency.
- Patient admission forecasting: AI analyzes health trends and historical data to predict patient influx, aiding in staff and resource planning
- Medical supply management: Accurate demand forecasts ensure essential supplies are available when needed, preventing shortages
- Staffing optimization: Predictive models help in scheduling medical personnel based on anticipated patient volumes, enhancing care quality
During the COVID-19 pandemic, governments and pharmaceutical companies used demand forecasting to anticipate vaccine needs worldwide. By analyzing infection rates, population density, and vaccine hesitancy data, supply chain managers optimized the distribution of doses
Three steps to get started with demand forecasting
Follow these three steps to establish strong demand forecasting practices and build a smarter, more resilient supply chain:
- Let demand forecasting do its job: Demand forecasting is the backbone of supply chain planning. Other supply chain functions, like S&OP, inventory optimization, and supply planning, simultaneously play a crucial role. When used correctly, these tools work together within an integrated system and deliver accurate predictions.
- Feed it the right data: AI-powered demand forecasting thrives on data. The more you feed it, the smarter it gets. Don’t just rely on past sales and historical trends. Expand your inputs to include market news, political shifts, social trends, and customer insights. Modern data management tools can process vast, complex datasets, and AI can turn these datasets into predictive analytics. Use these together to drive better decisions.
- Budget and plan for success: Demand forecasting isn’t just about technology. Strategy plays a pivotal role. Legacy systems and old workflows are tough to change and resistance is natural. Businesses can ease the transition with the right planning. Set aside budget and resources early. Get leadership buy-in. Make change management a priority. Use business intelligence to better understand the impact of your supply chain data.
What’s next for supply chains?
The future belongs to those who predict it. With demand forecasting, businesses make accurate predictions and keep up. This helps prevent waste, cut costs, and keep customers happy.
While traditional forecasting methods have served businesses well, they often fail to capture the complexities of today’s dynamic markets. AI-driven demand forecasting is changing the game, offering unparalleled accuracy, adaptability, and efficiency.
As businesses grow and supply chains get more complex, the winners will be those who embrace AI-driven forecasting to navigate uncertainty, streamline operations, and stay ahead.
Is AI good for demand forecasting?
What are the sources of data for demand forecasting?
What is an example of demand forecasting?
Building a data platform doesn’t have to be hectic. Spending over four months and 20% dev time just to set up your data platform is ridiculous. Make 5X your data partner with faster setups, lower upfront costs, and 0% dev time. Let your data engineering team focus on actioning insights, not building infrastructure ;)
Book a free consultationHere are some next steps you can take:
- Want to see it in action? Request a free demo.
- Want more guidance on using Preset via 5X? Explore our Help Docs.
- Ready to consolidate your data pipeline? Chat with us now.
Get notified when a new article is released
Get an end-to-end use case built in 48 hours
Get an end-to-end use case built in 48 hours
How retail leaders unlock hidden profits and 10% margins
Retailers are sitting on untapped profit opportunities—through pricing, inventory, and procurement. Find out how to uncover these hidden gains in our free webinar.
Save your spot
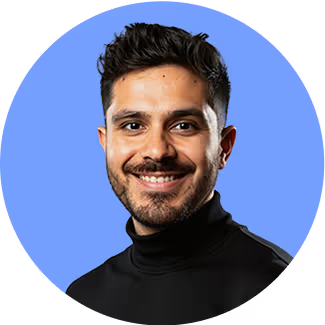