Data Trust Score: Measuring and Improving Data Reliability Across the Enterprise
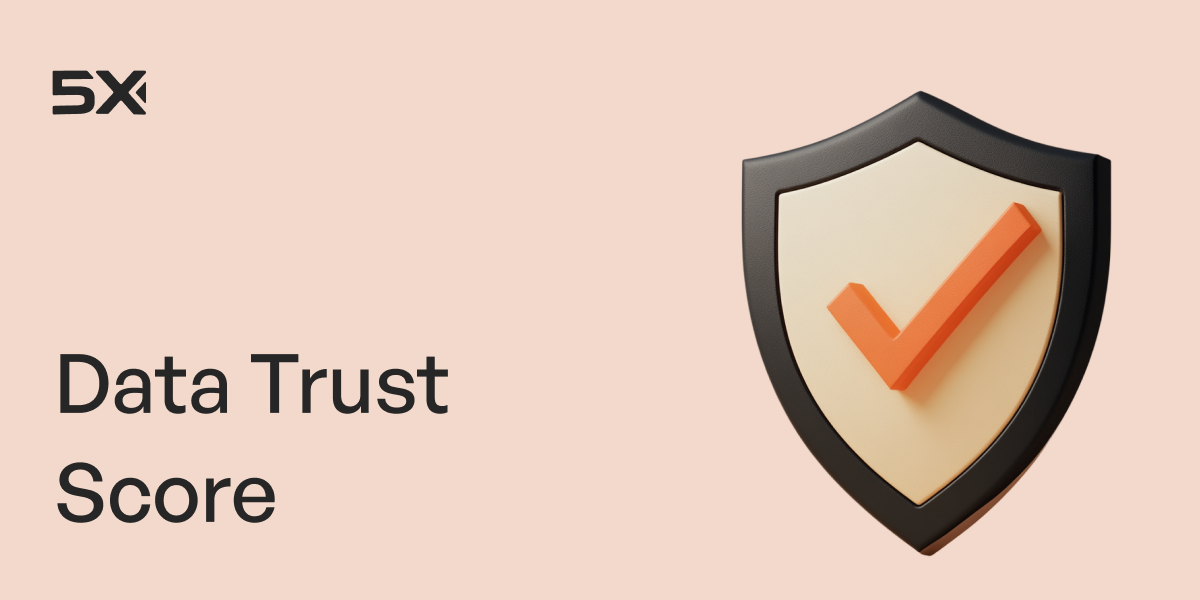
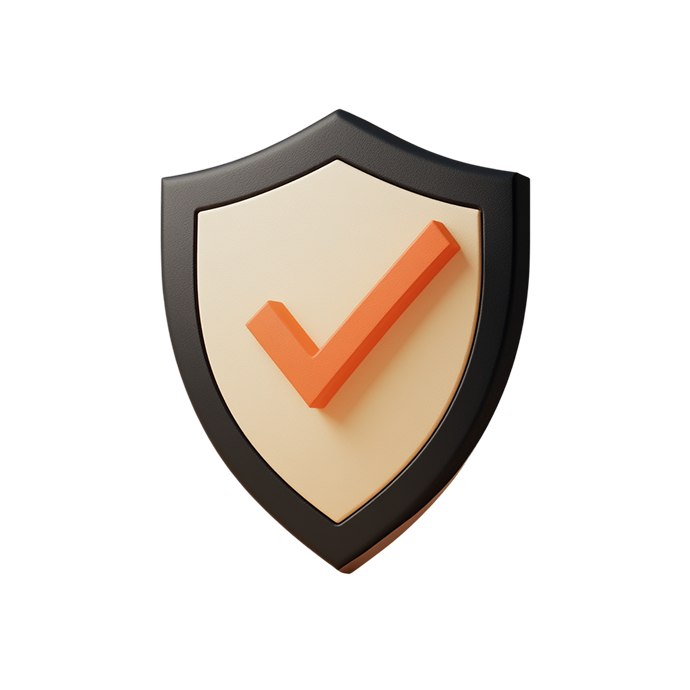
Table of Contents
Without confidence in your data’s accuracy, consistency, and origin, even the most advanced analytics and AI models can prove ineffective. Data trust score can bridge this gap.
Data Trust Score (DTS) is a structured way to quantify the reliability of a dataset, and has real-world applications and use cases across industries. Read this blog to learn about a data trust score, frameworks you can use, and how you can leverage it for business success.
By going from reactive data cleanup to proactive data trust, organizations can thrive in the data-driven economy of tomorrow. High-quality data allows AI models to learn from accurate patterns and make reliable predictions, translating to better organizational decision-making.
Vipin Jain, IT Strategist, Transformation Enablers
Source: CIO.com
What is data trust?
Data trust is the confidence an organization has in its data’s accuracy, reliability, and readiness for decision-making and operational use. In strong data-driven cultures with organizational agility, data trust enables “data health”, which is the foundation for effective business strategies.
Achieving data trust is not a one-time engagement, but a continuous process of ensuring that your data is reliable and fit for use. When data is trusted across departments, it empowers teams to:
- Design better customer experiences: Organizations that trust their data can make targeted product recommendations based on past purchases
- Make faster, more confident decisions: Make dynamic pricing based on real-time sales data
- Improve innovation: Use quality data for AI model training or product development
Trust in data must be earned through evidence, not assumed. This requires ongoing validation, quality checks, and transparency around data sources.
What is a data trust score (DSC) and how does it work?
Data trust score is calculated using numerical indicators that measure how reliable, accurate, and credible a dataset or data source is. It brings structure, accountability, and transparency to data quality management, enabling smarter, safer, and faster business decisions.
It is important because without a structured way to evaluate data quality, organizations risk making decisions based on outdated, incomplete, or inaccurate information.
By implementing a standardized trust scoring framework, organizations can:
- Assess data based on quality, source integrity, and relevance to business context
- Improve confidence in analytics and reporting
- Strengthen data governance practices and compliance
- Minimize the risks associated with poor data quality
Steps in the data scoring process
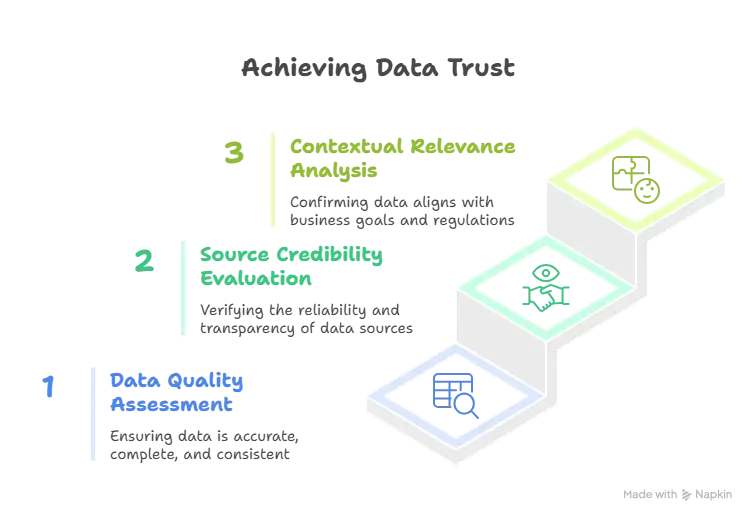
To determine how much your organization can trust a given dataset, a structured scoring process is followed. This process typically includes three key steps:
Step 1: Data quality assessment
This is the foundation of any trust score. This step checks if the data is correct, complete, and consistent, and evaluates how well the data meets technical and business expectations.
To assess data quality, check:
- Accuracy: It’s about how well your data reflects the real-world value it is intended to represent. It is measured through validation checks, error rates, or cross-verification with authoritative sources.
- Example: If a customer’s age is listed as 180 or their city as “123,” data is inaccurate and cannot be trusted
- Example: If a customer’s age is listed as 180 or their city as “123,” data is inaccurate and cannot be trusted
- Completeness: If all required data fields in your dataset are filled and nothing essential is missing, your dataset is complete. Completeness is measured based on the percentage of missing or null values in important fields
- Example: A sales record should have customer name, product ID, quantity, transaction date and any other information that’s relevant to sales
- Example: A sales record should have customer name, product ID, quantity, transaction date and any other information that’s relevant to sales
- Consistency: The same data should be consistent across different systems and reports. It is measured by comparing data across systems and checking for conflicts
- Example: A product’s price should match in the inventory database and on the sales portal. Similarly, a customer’s address must be uniform in the CRM and billing system
- Example: A product’s price should match in the inventory database and on the sales portal. Similarly, a customer’s address must be uniform in the CRM and billing system
Step 2: Source credibility evaluation
With reliable sources comes the confidence that data can be used without risks. Evaluating source credibility is crucial because even high-quality data can be misleading if it comes from an unreliable or unverified source. This stage focuses on evaluating the origin of the data.
For source credibility analysis, check:
- Reputation of the source: Is the data generated by a reliable internal system, a trusted third-party provider, or an unverified source?
- Example: Data from a government health agency will generally score higher than data scraped from an unknown website.
- Example: Data from a government health agency will generally score higher than data scraped from an unknown website.
- Data history: Has the source provided accurate and reliable data over time?
- Example: A supplier that regularly uploads incorrect shipment logs may be flagged as unreliable
- Example: A supplier that regularly uploads incorrect shipment logs may be flagged as unreliable
- Transparency: This is about clearly tracing the origin and collection process of the data. Is the method of data collection well-documented and auditable? Can the source provide metadata or logs to verify authenticity?
- Example: IoT sensor data with time stamped logs will score higher than undocumented spreadsheets
- Example: IoT sensor data with time stamped logs will score higher than undocumented spreadsheets
- Bias and manipulation risk: Does the data source have a potential conflict of interest or motive to skew the data.
- Example: Product reviews from a brand's internal team may carry bias compared to independent reviews
- Example: Product reviews from a brand's internal team may carry bias compared to independent reviews
Step 3: Contextual relevance analysis
High-quality, credible data may still not be suitable if it’s not relevant to the specific decision or context it’s being used in. This step ensures that data is fit-for-purpose.
To analyze contextual relevance, check:
- Alignment with business goals: Does the data help answer the business question at hand?
- Example: Sales forecasting requires historical sales trends, not customer satisfaction scores. Scoring data trust on the latter will not work
- Example: Sales forecasting requires historical sales trends, not customer satisfaction scores. Scoring data trust on the latter will not work
- Granularity and scope: Is the data detailed or aggregated at the right level?
- Example: Product-level returns data is more useful than overall monthly return rates when diagnosing quality issues
- Example: Product-level returns data is more useful than overall monthly return rates when diagnosing quality issues
- Time relevance: Is the data recent enough to reflect current market or operational conditions? How up-to-date the data is relative to its intended use?
- Example: A 3-year-old customer survey may be irrelevant for today's product strategy. Data must be fresh and the last 6-12 must be considered
- Example: A 3-year-old customer survey may be irrelevant for today's product strategy. Data must be fresh and the last 6-12 must be considered
- Regulatory and operational context: Is the data collected and used in compliance with regulations and business policies?
- Example: Using customer data without proper consent violates data privacy laws, regardless of quality, making it tough to trust your data
What’s a data trust framework and how does it work?

The Data Trust Framework is a structured set of principles, practices, and tools that guide how organizations manage and protect their data. Its primary goal is helping businesses establish confidence in the reliability, integrity, and ethical use of their data, both internally and externally.
The framework ensures that data trust is built deliberately through good governance, ethical practices, and transparent systems. Key components include:
- Data governance policies: These are formal rules and procedures that determine how data is collected, stored, accessed, shared, and retired. It includes:
- Data ownership and stewardship roles (Who is responsible for what?)
- Data classification policies (What data is sensitive or regulated?)
- Data lifecycle management (When is data archived or deleted?)
- Compliance protocols with regulatory standards (e.g., GDPR, SOC 2, CCPA)
- Example: A financial institution may implement strict policies on how customer data is encrypted, who can access it, and how long it's retained
- Ethical guidelines: Ethics in data goes beyond compliance to address how data should be used responsibly and with respect for individuals' rights. It includes:
- Informed consent for data collection and usage
- Transparency on how data will be used
- Bias mitigation in AI/ML models
- Fairness in automated decision-making
- Example: An e-commerce platform clearly communicates that it uses customer browsing data to recommend products, and gives users the option to opt out
- Accountability measures: These mechanisms ensure that the policies and guidelines are followed, and that there are consequences when they are not. It includes:
- Audit trails and logging systems to track who accessed or changed data
- Performance metrics for data quality and compliance
- Data incident response plans
- Internal and external audits
- Example: A healthcare company runs quarterly audits to ensure patient data is only accessed by authorized personnel and has detailed logs of every access
What are the real world applications of a data trust score?

Data trust scores have practical applications across industries and help organizations reduce risk, ensure compliance, and improve the quality of decision-making.
Key industry applications include:
1. Healthcare: Ensuring patient safety and treatment accuracy
In the healthcare sector, the accuracy and reliability of patient data are directly tied to patient outcomes and safety. A hospital may use Data Trust Scores to assess whether a patient’s electronic health record (EHR) is complete and accurate before a critical surgery.
Use cases:
- Verifying the integrity of patient medical histories before prescribing medication
- Evaluating the reliability of external research or clinical trial data used in diagnosis or treatment planning
- Ensuring that real-time data collected from wearable health devices meets acceptable standards for clinical decision-making
2. Finance: Managing risk and strengthening compliance
Financial institutions rely heavily on data to forecast market trends, assess client risk, and ensure compliance with regulations. Trust scores help ensure that decisions are based on credible data sources. For example, a bank might score customer transaction data to determine whether it’s reliable enough to feed into a machine-learning model that predicts credit default risk.
Use cases:
- Assessing the trustworthiness of third-party market intelligence before making or recommending investment decisions to customers
- Evaluating internal credit score models and ensuring customer financial data is complete and consistent to ensure that decisions are not premature
- Auditing financial transactions to flag anomalies or discrepancies in large datasets
3. Marketing: Targeting the right audience with confidence
Marketing departments use customer and behavioral data to tailor campaigns. Data trust scores help validate this data, so that campaigns are based on credible insights and lead to better ROI. For example, an e-commerce brand may only use customer profiles with high Trust Scores for personalized promotions, reducing the chance of wasted ad spend or messaging errors.
Use cases:
- Scoring lead data for accuracy and freshness before launching an email campaign
- Evaluating the reliability of social media listening tools and their output
- Assessing customer segmentation models to ensure the underlying data is consistent and up to date
4. Supply chain & logistics: Improving forecasting and efficiency
Operational teams use data trust scores to assess inventory data, supplier reliability, and logistics performance, allowing for better planning and risk mitigation. A retailer often uses trust scores to flag inconsistencies in stock level data across warehouses, ensuring accurate demand forecasting.
Use Cases:
- Validating inventory levels before automated reorder decisions
- Scoring supplier data to identify inconsistencies in delivery timelines or quality metrics
- Evaluating transportation data to forecast delays and reroute shipments
5. Public sector & policy: Supporting evidence-based governance
Governments and NGOs rely on massive datasets to design and implement policies. Trust Scores ensure that decisions are based on credible and unbiased information. A municipal government may use trust scores to validate data from a public transport survey before investing in infrastructure.
Use Cases:
- Assessing census or demographic data before designing public health or education programs
- Scoring third-party research used in policy recommendations
- Validating citizen feedback data to inform smart city development projects
Turn data into a competitive advantage with 5X
5X allows you to easily centralize and streamline your data operations by unifying data into a single, actionable platform. Using 5X, you can implement and monitor data trust scores and ensure that your data remains a reliable foundation for strategic initiatives.
FAQs
Why is a data trust score important for enterprises?
How is a data trust score calculated?
Building a data platform doesn’t have to be hectic. Spending over four months and 20% dev time just to set up your data platform is ridiculous. Make 5X your data partner with faster setups, lower upfront costs, and 0% dev time. Let your data engineering team focus on actioning insights, not building infrastructure ;)
Book a free consultationHere are some next steps you can take:
- Want to see it in action? Request a free demo.
- Want more guidance on using Preset via 5X? Explore our Help Docs.
- Ready to consolidate your data pipeline? Chat with us now.
Get notified when a new article is released
Get an end-to-end use case built in 48 hours
Get an end-to-end use case built in 48 hours
How retail leaders unlock hidden profits and 10% margins
Retailers are sitting on untapped profit opportunities—through pricing, inventory, and procurement. Find out how to uncover these hidden gains in our free webinar.
Save your spot
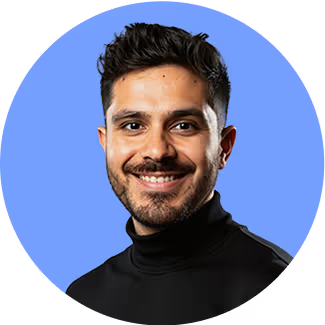