How AI is Revolutionizing Energy Consumption Forecasting in Manufacturing

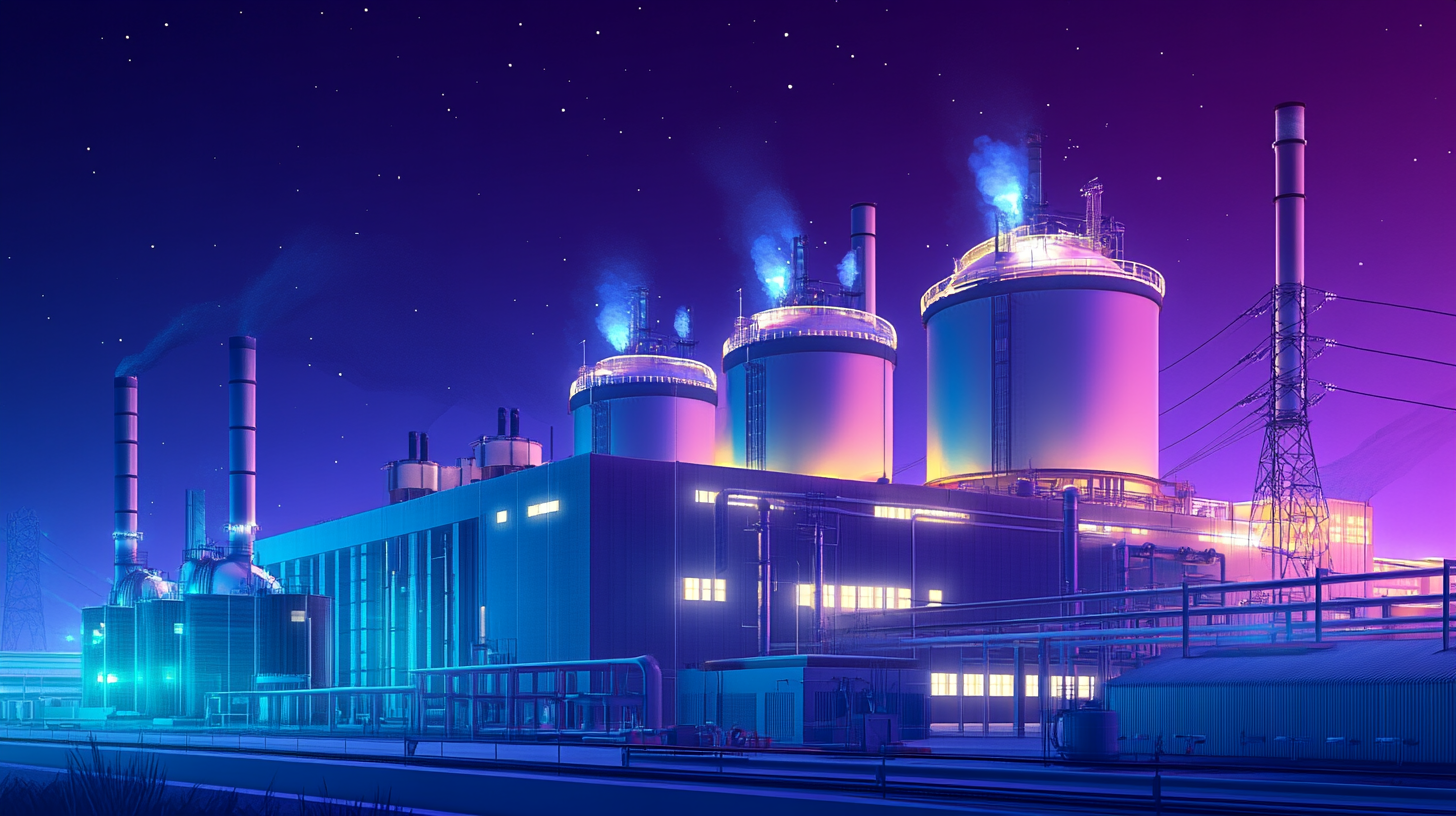
Table of Contents
Global pressure on energy efficiency is intensifying.
The Paris Agreement calls for a 43% reduction in global emissions by 2030. The Science Based Targets initiative (SBTi) now expects manufacturing companies to show measurable decarbonization paths. And with over 4,000 companies signed on to meet these science-based goals, the question is no longer if industry should change, it's how fast.
Energy consumption is one of the biggest culprits. Industrial players account for over 37% of global energy use. But the bigger issue? Most still run on outdated energy management strategies like manual logging, reactive decisions, and guesswork.
Rising energy prices and unpredictable grid demands have turned inefficiency into a business risk. You overspend. You miss ESG goals. You lose competitive ground.
That’s where AI forecasting comes in.
With AI, you don’t wait for your next utility bill to discover you’ve overused. You get live, data-backed forecasts that help you adjust in real time. You cut waste before it happens. You plan ahead with confidence, not spreadsheets.
This post breaks down exactly how AI helps industries forecast energy use with precision, reduce operational costs, and meet aggressive sustainability targets, without slowing down growth.
What is AI forecasting in energy consumption?
AI forecasting in energy consumption uses artificial intelligence to predict how much energy will be needed in the future. It looks at past data, weather forecasts, and real-time inputs to give businesses a clear picture of their energy needs.
- AI learns from historical energy data and patterns from past consumption help predict future needs
- It also tracks weather conditions, as temperature changes affect energy use
- Operational data plays a role too: shifts in production, machine use, or occupancy all impact energy demand
For instance, in manufacturing, AI predicts when machines will consume more power, such as during peak hours or specific processes. Manufacturers can then schedule energy use wisely, reducing costs and waste.
In smart buildings, AI could help adjust heating, cooling, and lighting based on occupancy and weather conditions, ensuring energy is used only when needed.
How do machine learning and AI forecast energy consumption?

Machine learning (ML) looks at historical data, in this case, your past energy consumption patterns. It identifies trends: when usage spikes, when it drops, and how external factors, like weather, play a role. Once it has this data, it predicts when your energy demand will increase, allowing you to prepare in advance.
Neural networks take this further by analyzing more complex data, like fluctuations in temperature or changes in production schedules. They’re an advanced pattern-matching system, so they continuously learn and adjust, ensuring that your forecast is updated in real time.
Data-driven models rely on both historical and live data. For example, weather conditions or a change in production schedules can immediately impact energy usage. These models react instantly to shifts, refining predictions as new data comes in.
And this tech has already found its way in leading manufacturing companies. Take the case of DeepMind’s project with Google’s data centers. By feeding AI past data on energy use and real-time temperature data, DeepMind’s system now predicts the exact amount of energy needed for cooling. The result? 40% less energy spent on cooling alone.
3 Ways AI forecasting helps tackle energy inefficiencies
AI forecasting makes energy waste visible. It spots exactly where your systems leak energy—and how you can fix it.
- Energy waste reduction: Factories waste energy every day without even noticing. AI changes that. It scans energy usage patterns around the clock, pointing out when machines are running unnecessarily
- Optimizing equipment and system performance: Predictive maintenance powered by AI helps in anticipating equipment failures before they occur, ensuring systems operate at peak efficiency
- Long-term sustainability: AI aids in strategic planning by analyzing long-term energy usage trends, facilitating a shift towards renewable resources
3 Steps to using AI to forecast energy consumption
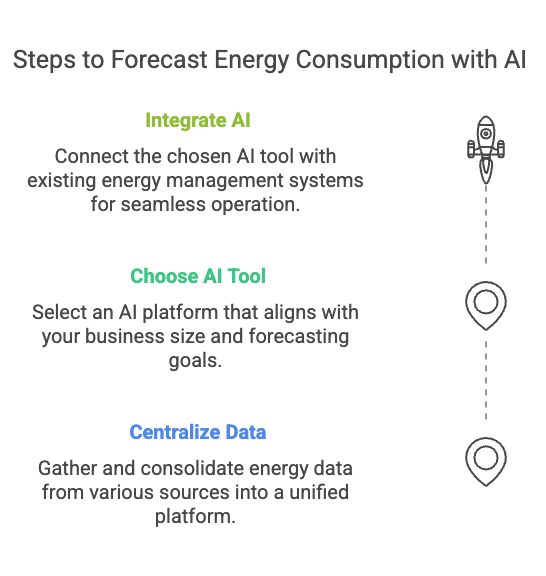
Forecasting your energy consumption with AI sounds complicated—it's not. You don't need a robot army or a room full of data scientists. Here's exactly how you can start, step-by-step:
Step #1: Get your data in one place
First, identify your sources of energy data. This might include meter readings, equipment sensors, or weather forecasts. But scattered data equals scattered results.
This is exactly where 5X steps in.
5X centralizes and consolidates your data effortlessly. With 600+ connectors, 5X pulls in data from practically anywhere, whether it's your factory sensors or external weather feeds. You get your data neatly stored so you can model and orchestrate it directly on 5X itself.
Want to deploy 5X on your existing cloud? Done. Prefer a managed software solution? Easy. Need integration with Snowflake or other data warehouses? Absolutely possible.
Also read: Top 6 Data Modeling Tools of 2025: Expert picks
Step #2: Choose your AI tool
Picking an AI tool is like picking coffee—specific and important for productivity.
Popular AI platforms include:
- Google Cloud AI Platform: Easy interface, ideal for predictive analytics
- Microsoft Azure Machine Learning: Robust and integrates smoothly with existing systems
- AWS Forecast: Specifically designed for resource forecasting
Action steps:
- Clarify your business size and forecasting goals before choosing
- Test-run a simple forecasting model as a pilot
Step #3: Integrate AI into your existing systems
Your new AI tools shouldn’t feel like outsiders. Connect them with your existing energy management systems through APIs or integrations.
Action steps:
- Link your AI platform with your energy dashboards or control systems
- Monitor regularly during the initial setup phase
Best practices to keep things smooth:
- Data collection: Automate as much as possible; avoid manual entry
- Data cleaning: Regularly audit your data, using tools like OpenRefine
- Continuous learning: Retrain AI models regularly as consumption patterns evolve
Start simple, stay consistent, and scale up gradually.
4 Challenges of implementing AI forecasting for energy consumption—and how to overcome them
AI forecasting is powerful, but it’s not plug-and-play. There are real challenges that slow down adoption, especially in traditional industries.
#1 Data quality and availability
AI is only as smart as the data you feed it. Inaccurate, incomplete, or outdated energy data leads to bad predictions. Unfortunately, many companies still rely on manual logs or scattered spreadsheets.
How to solve for data quality?
Start with a data audit. Identify gaps, errors, and inconsistencies. Use tools like dbt or Great Expectations to build data quality checks. And if you want to skip the setup headache, 5X can automate this for you. Clean, validated data is part of the foundation.
Also read: Data Quality Framework: Definition, Benefits, and Implementation
#2 Dealing with data silos
Your energy data probably lives across multiple systems, like meter logs, building management tools, production systems, ERP platforms. If AI can’t see the full picture, it can’t forecast accurately.
How to solve for data silos?
Centralize your data. Use 5X’s 600+ connectors to pull data from everywhere into a single source of truth. Then model, orchestrate, and deploy from one place. No patchwork integrations needed.
#3 Overcoming resistance to change
Not everyone loves “new.” Operators may be skeptical. Managers may hesitate to shift from their gut-based forecasts.
How to solve for resistance?
Start with a small, visible use case. Let the AI prove itself. Show the team how it saved energy or predicted peak usage. And invest in training. Once people see the benefits, buy-in follows.
#4 Cost of implementation
AI can feel expensive, especially for smaller operations.
How to solve for costs?
Skip the 6-month data science build. Use ready-made, scalable platforms like 5X, Google Cloud, or AWS Forecast. You don’t need a data team—you need clean data and a good interface.
Forecast less. Know more. Act faster.
AI forecasting helps you cut waste, plan smarter, and make energy decisions backed by data, not guesswork. From identifying inefficiencies to predicting future demand, the results are tangible: lower costs, better resource planning, and progress toward sustainability goals.
If you’re serious about reducing energy waste and running a tighter operation, now’s the time to act. Start with a clear view of your data. Choose the right AI tools. Run a focused pilot. Improve, expand, repeat.
You don’t need to build from scratch. Platforms like 5X give you a fast, integrated way to centralize your energy data, run AI models, and see real results—without needing a data team.
FAQs
What is the forecast for AI energy consumption?
How is AI used in the energy industry?
How can AI reduce energy consumption?
How can AI be used in forecasting?
Building a data platform doesn’t have to be hectic. Spending over four months and 20% dev time just to set up your data platform is ridiculous. Make 5X your data partner with faster setups, lower upfront costs, and 0% dev time. Let your data engineering team focus on actioning insights, not building infrastructure ;)
Book a free consultationHere are some next steps you can take:
- Want to see it in action? Request a free demo.
- Want more guidance on using Preset via 5X? Explore our Help Docs.
- Ready to consolidate your data pipeline? Chat with us now.
Get notified when a new article is released
Get an end-to-end use case built in 48 hours
Get an end-to-end use case built in 48 hours
How retail leaders unlock hidden profits and 10% margins
Retailers are sitting on untapped profit opportunities—through pricing, inventory, and procurement. Find out how to uncover these hidden gains in our free webinar.
Save your spot
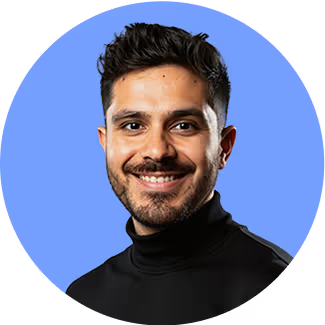